How Artificial Intelligence in Cyber Security Actually Works: Myths vs Reality
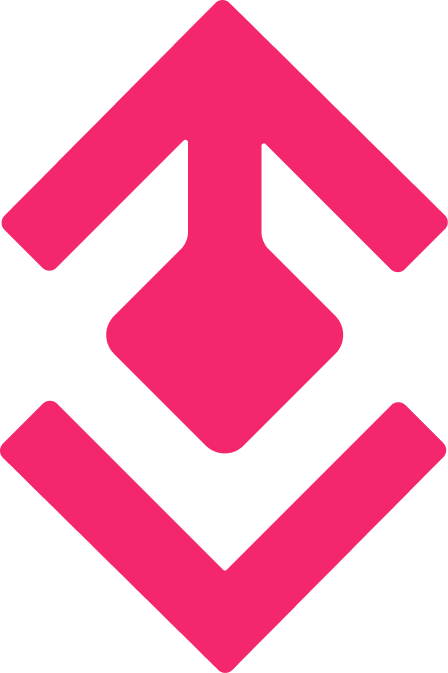
The artificial intelligence cybersecurity market shows explosive growth. Experts project a jump in revenue from $22.4 billion in 2023 to $60.6 billion by 2028. This surge makes sense as 86% of organizations have stepped up their use of AI security tools by a lot in the last year.
However, while AI cybersecurity solutions promise better threat detection and automated responses, actual capabilities are different from what most people think. These systems excel at specific tasks: they analyze big datasets to spot threats, detect unusual user behavior, and respond automatically to security problems. However, they have many limitations.
This piece strips away the hype about AI security tools' capabilities. You'll find the truth behind popular myths and see how AI works with human security teams to shield organizations from emerging cyber threats.
Common Myths About AI in Cybersecurity
People's misconceptions about artificial intelligence in cyber security shape how organizations make decisions and plan their security strategies. These myths create unrealistic expectations and make companies hesitant to adopt AI security solutions.
Myth #1: AI Will Replace Human Security Teams
Many worry about AI taking over, but it actually works as a powerful assistant for security professionals. Research shows that AI does well with automating routine tasks and handles large datasets. This lets human experts concentrate on making strategic decisions and solving complex problems. The emergence of new roles like prompt engineers shows how AI creates job opportunities instead of eliminating them.
The truth is, teams get better results when they combine human expertise with AI capabilities. Human judgment plays a vital role in interpreting AI findings, handle false alarms, and make final security decisions. The core team provides oversight that sets objectives and makes sure AI systems match the organization's security goals.
Myth #2: AI Systems Are Infallible
The idea that AI security systems never make mistakes ignores their limitations. These systems can trigger false alarms and sometimes miss threats, especially when they face new attack vectors absent from their training data. The quality and variety of training data affects AI solutions, which makes them prone to biases and errors.
Cybercriminals keep developing new tactics, and AI systems aren't immune to these evolving threats. Research shows that adversaries can trick AI models into misclassifying malware, which highlights why we need to keep refining these systems.
Myth #3: AI Security Solutions Are Too Complex to Implement
Many organizations think AI security solutions need extensive technical expertise or substantial resources. All the same, modern AI security tools have become more user-friendly and available. Cloud-based solutions now let businesses of all sizes use AI security measures without spending too much.
This is not to say that implementing AI is simple, though; there are some challenges. Integration challenges come from:
- The need to customize for specific network environments
- Requirements for ongoing monitoring and updates
- Staff training and education needs
A financial institution's story shows this reality - their original AI-based intrusion detection system triggered many false alarms until they properly adjusted it to their network environment. The system's accuracy improved substantially afterward, which shows successful implementation needs proper configuration, not just complex technical knowledge.
The Reality of AI Threat Detection
AI in cybersecurity goes beyond myths and misconceptions. It works through sophisticated pattern recognition and behavioral analysis systems. Security teams now utilize AI to process massive amounts of data and spot potential threats faster than ever before.
How AI Actually Identifies Threats
AI threat detection systems look at network traffic, user behavior, and system activity in real-time. Machine learning algorithms establish baseline patterns of normal operations and flag any deviations that might pose security risks. Though the use of non-stop monitoring, AI spots suspicious patterns that could signal a breach or attack.
AI detection's main strength comes from processing huge amounts of data. Systems powered by artificial intelligence can analyze multiple data streams at once, including:
- Network traffic patterns and anomalies
- User access behaviors and authentication attempts
- System log files and configuration changes
- Email metadata and communication patterns
Limitations of AI Detection Systems
AI-based threat detection is powerful, but it has its limits. These systems depend heavily on their training data's quality and variety. Even the best-trained AI models can trigger false alarms and need constant fine-tuning to stay accurate.
AI systems can't catch completely new attack patterns they haven't seen before, and smart cybercriminals often design attacks that slip past AI detection by copying normal behavior patterns. On top of that, some AI algorithms work like "black boxes," making it difficult for security teams to understand why certain threats get flagged.
Human-AI Collaboration in Threat Detection
The best results come from combining AI's analytical power with human expertise. Security analysts watch over the process, make sense of AI alerts, and decide what to do about potential threats. This team effort lets organizations use both AI's processing power and human intuition effectively.
AI handles the first round of threat detection and sorting. It processes vast amounts of data to spot possible security incidents. Security teams then take a closer look at these alerts and use their knowledge to decide how to respond. This approach works especially well against sophisticated attacks that automated systems might miss, and this relationship only strengthens as time goes on.
AI systems learn from security team feedback, while analysts can focus on complex cases instead of routine monitoring. This partnership keeps getting stronger, bringing together AI's speed and pattern recognition with human judgment and strategic thinking.
Truth About AI-Powered Security Automation
AI-powered security automation represents a major step forward in how organizations deal with cyber threats. Companies that use AI-powered security automation save 65.2% on total breach costs.
Ground Capabilities of Automated Response
AI security automation shines at quick threat detection and response by analyzing big datasets in real-time. These systems can isolate compromised systems, block malicious activities, and reset compromised credentials without human input.
Automated response systems can handle:
- Automated vulnerability assessment and patch management
- Real-time network traffic monitoring and analysis
- Instant threat containment and system isolation
- Automated compliance monitoring and reporting
In fact, AI cyber security systems can process data from various sources. They analyze network traffic, user behavior, and external threat feeds to spot potential threats quickly and accurately.
Where Automation Falls Short
So, while automation brings many benefits, it has its limits. False positives and negatives pose a major challenge. These can make security teams less responsive if they happen too often. AI systems also lack human intuition and context needed to review how important specific alerts are.
Security teams then face problems when automated systems create too much data. This can overwhelm analysts if they don't manage it well, and alert fatigue is a very, very real thing. Organizations must balance automation with human oversight to ensure their threat response works.
Integration with Existing Security Systems
AI-powered security automation needs smooth integration with current security systems. Organizations should check if AI tools work with their existing setup. This includes Security Information and Event Management (SIEM) platforms, firewalls, and intrusion detection systems.
The integration needs careful planning and several key steps. Organizations should first check their security setup and find where AI helps most. Security teams must also ensure automated systems talk to existing tools through standard protocols and APIs.
A step-by-step approach works best for successful integration. Teams can start with specific tasks, like threat detection or incident response, before moving to other areas. This careful approach helps organizations keep running smoothly while improving their security.
Integrated AI security systems need constant attention and updates. Security teams should test and update AI models regularly to stay effective as threats change. Organizations also need strict access controls and privacy tools to protect sensitive data that AI systems process.
Debunking AI Privacy Concerns
Privacy concerns about artificial intelligence in cyber security have grown as data collection keeps expanding. Look at ChatGPT - its training dataset jumped from 1.5 billion parameters in 2019 to 175 billion parameters in 2020. These numbers show just how big data requirements have become in modern AI systems.
Data Collection
AI security systems are built on large datasets gathered from multiple sources. A few of the sources include:
- Website interactions and browsing patterns
- Social media activity and user priorities
- Biometric data including facial recognition
- Geolocation and movement tracking
- Consumer transaction records
- Device usage patterns
This massive data collection creates serious privacy issues. AI systems can leak personal information through pattern analysis and data correlation during normal operations. Even anonymized datasets are risky. AI algorithms can figure out individual identities by combining multiple data sources or tracking specific data points over time.
Privacy challenges go beyond basic data gathering. AI systems look for patterns in collected information and create detailed profiles from seemingly unconnected data points. A data broker could learn private details about income, religion, relationship status, or political affiliations just by analyzing shopping history and internet browsing patterns.
The reach of potential privacy breaches has grown by a lot. AI systems can now track people through mobile location data. They create detailed life patterns that could identify specific individuals from otherwise anonymous devices. These systems also enable unprecedented surveillance levels, bringing new concerns about biometric identification and immediate social media tracking.
Of course, privacy breaches have major economic impact. Companies use AI to analyze customer behavior for pricing decisions. To cite an instance, General Motors sold information about customers' trip lengths, speed, and driving habits to data brokers, which affected insurance premium calculations.
Data protection becomes trickier as AI systems evolve. Organizations need to balance productivity gains against privacy risks. Machine learning models don't deal very well with tracing data sources. This makes it hard to follow privacy laws, especially when handling data subject rights like access or erasure requests.
Organizations should use strict data minimization practices to handle these issues. They need to limit data collection to what's legally allowed and set clear timelines for data retention. Companies must also give people ways to consent, access, and control their personal data. This ensures transparency in how information gets collected and used.
In short AI in cyber security has its definite place and advantages. However, it does have drawbacks that must be addressed and solved, even as the security (and AI) landscapes change.
FAQs
Q1. How does AI actually function in cybersecurity? AI in cybersecurity uses machine learning algorithms to analyze vast amounts of data in real-time. It establishes baseline patterns of normal operations and flags deviations as potential security risks. AI systems monitor network traffic, user behavior, and system activity to identify suspicious patterns that might indicate a breach or attack.
Q2. Can AI completely replace human security teams? No, AI cannot completely replace human security teams. Instead, it serves as a powerful assistant, automating routine tasks and analyzing large datasets. Human expertise remains crucial for interpreting AI findings, resolving false alarms, and making final security decisions. The most effective approach combines AI's analytical capabilities with human judgment and strategic thinking.
Q3. What are the limitations of AI in cybersecurity? AI systems in cybersecurity have several limitations. They heavily depend on the quality and diversity of their training data and can generate false positives. AI models struggle with completely novel attack patterns not present in their training data. Additionally, sophisticated cybercriminals can design attacks to evade AI detection by mimicking normal behavior patterns.
Q4. How does AI-powered security automation benefit organizations? AI-powered security automation offers rapid threat detection and response capabilities. It can automatically isolate compromised systems, block malicious activities, and reset compromised credentials without human intervention. Organizations using AI-powered security automation can save significantly on total breach costs and improve their overall security posture.
Q5. What privacy concerns arise from AI use in cybersecurity? AI in cybersecurity raises privacy concerns due to its extensive data collection and analysis capabilities. These systems can unintentionally expose personal information through pattern analysis and data correlation. Even anonymized datasets pose risks, as AI algorithms can deduce individual identities by combining multiple data sources. Organizations must balance productivity gains against privacy risks and implement strict data protection measures.